Unlock ChatGPT's Potential: The Art of Asking Great Questions.
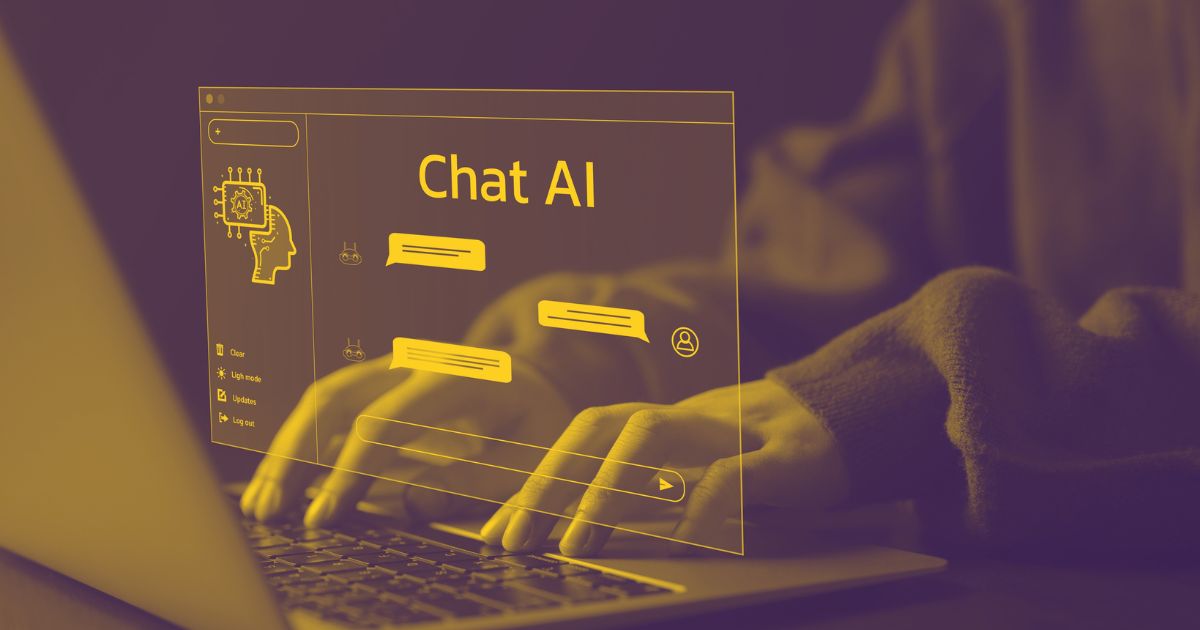
- April 16, 2024
- 1223
There’s no escaping ChatGPT and the Generative AI (GenAI) movement—err, revolution. The future is here, and it’s becoming more evenly distributed. Or is it? I’m almost fatigued by the nonstop content purporting new GenAI offerings, ChatGPT, Midjourney, or DALL-E lookalikes, and doomsday or overly optimistic AI predictions. I’m left questioning the motivations driving this content hype train. And yet, here I am, contributing yet another perspective. However, I want to take a step back and evaluate where we’re at, where we’re going, and what we should be thinking about today to be ready for tomorrow. From my vantage point, we aren’t asking the right questions.
Examining the Gartner Hype CycleTM reveals that cycles are common in emerging technology. The technology trigger is the first step in the process, which is then followed by the Peak of Inflated Expectations, the Trough of Disillusionment, the Recovery to the Slope of Enlightenment, and the Plateau of Productivity.
Where in the hype cycle do you think GenAI is at the moment?
We're yelling to the top of the Peak of Inflated Expectations, in my opinion. Although there is still much to be done, there is a lot of promise with GenAI. As we continue to run into its real and perceived limitations, we will discover that we are still in search of the capabilities that will allow us to achieve significant productivity increases. That's a strong statement, so allow me to explain that observation.
In his book Zero to One, Peter Thiel makes the case that successful companies are the result of outperforming their rivals ten times. Although generative AI has the potential to be ten times more powerful than it currently is, no firm has yet to outperform me. Although many businesses are travelling the same path and developing competitive GenAI capabilities, OpenAI has made progress. The neural network and backpropagation research that Geoffrey Hinton and others have been advancing for decades form the basis of generative artificial intelligence. But because of the cloud, the massive resources needed to train models with billions of parameters are now easily accessible; the main limitation is funding these resources.
Stated differently, some of the vision has only recently begun to materialise despite decades of advancements in technology and know-how. The ingenuity of the individual posing the question, however, currently limits the models' potential. I don't think this is a ten-fold improvement. For my lovers of AutoGPT, it's not a tenfold improvement, but it is a tiny step.
Where are we, then, if we haven't reached the 10x capability yet?
I recently looked over the GenAI use cases generated by a reputable technological advisory firm. Each use case required a human engineer to create an initial prompt. Even though GenAI's results from these prompts are astounding, there are still certain issues that need to be resolved. Even worse, discussions on whether or not people should switch from studying STEM (science, technology, engineering, and math) to language arts in order to become more proficient engineers are being sparked by this GenAI limitation. I will revisit this.
Let's begin with confidence. One of the greatest disclaimers for content generated by GenAI, perhaps. It is advised that we "trust but verify." The media at large attributes the cause to "hallucinations." Hinton, however, points out that they are known as confabulations—the propensity to fabricate or generate false memories without intending to mislead. Hinton also points out that human beings act in similar ways. Have you ever experienced these erroneous memories? Thus, we must verify the information that GenAI so boldly provides. This validation may also take longer than it would have if we had created the original content ourselves, depending on the content that has been provided.
How are you balancing the use of GenAI to accelerate content generation?
For more than ten years, semi-autonomous cars have been driving on the road, sending data to headquarters to enhance the models that power the semi-autonomous mode. Furthermore, people still need to stay focused on the road. My argument is that while output accuracy will continue to improve, mistakes can significantly increase over greater distances with a little one-degree divergence. Thus, be prepared to devote time and effort to validation and improvement.
The second item on the list is contextual memory. Big Language Models gain from having a corpus that encompasses a large portion of human knowledge. I'll put the copyright debate on hold for the time being in order to address another issue. What is GenAI's contextual memory? Anybody who has used ChatGPT for an extended conversation or question would have encountered the enforced character restriction. ChatGPT eventually loses track of the entire context of your exchange and the data you're exchanging. The rationale for this stems from the way these models function and the amount of processing power required to carry out the workflow that converts the input into numerical representations, which are subsequently used to forecast which words or pixels to display to you. As a result, because of its limited memory, GenAI is now only capable of handling problems that are relatively simple to solve in one sitting. I think improvements will occur sooner rather than later. Nonetheless, humans must still be adept at comprehending intricate systems and able to disassemble larger systems into smaller parts so that GenAI can handle content production in an efficient manner. These abilities go beyond the mastery of prompt engineering.
It would be negligent of me to ignore security. Sensitive data exposure to these online GenAI models—which are subsequently utilised to train new iterations of the model—is the current cause for concern. Your contribution might wind up serving as a response to a future question from someone else. Consider information to be the secret ingredient of your company. Creating walled gardens where the data you provide is isolated from the data needed to train the GenAI model is the best short-term solution. Furthermore, methods based on the so-called embedded model are beginning to emerge. These enable you to train your own models with your data and have ChatGPT programmatically communicate with the model in order to gather input and subsequently transform it into a human-understandable response. Hardly ideal, since the model is trained in a way that does not maintain the original file, record, etc., making the policy-driven security models that are now in use less efficient. My prediction is that security will go from policy-driven security models to context-driven security models, where security is applied based on context instead of explicit regulations associated with resources, files, folders, and records.
Now, back to my "wrong questions" assertion. In the event that the queries we are posing are incorrect, what inquiries do we need to make? Let's pose the incorrect queries first. The excessively prevalent zero-sum game mentality of the internet might be difficult to ignore. Because it makes for compelling clickbait, the first thing that comes to mind is whether GenAI will eventually replace humans. It's not the right question. The relationship between people and technology requires a certain amount of synthesis, balance, and equilibrium. Because humans may conjure up new futures by using their innate creative abilities, this equilibrium is constantly shifting. As those aspirations come true, we adapt and transform our relationship with technology. The proper query is: How can we rebalance our system to optimise the benefits of our collaboration between humans and GenAI? How can we investigate improving human augmentation? Using GenAI to reduce costs by eliminating human labour is not a 10x idea. The issue of replacing humans highlights a lack of understanding of the larger system because reducing costs is frequently a short-term benefit that results in a longer-term decline in competitiveness. Humans are undoubtedly capable of greater creativity.
AI has been hailed as the next big thing for a long time. The future is now more widely spread, or at the very least acknowledged, as the next revolution because of ChatGPT and GenAI. I suggest you think beyond the first use cases and do what people do best: imagine a creative future in which GenAI works alongside people rather than as a substitute for them. This imagined future will come to pass, but will it come to you in equal measure?